Inclusive AI - in the age of racial discrimination
Updated: Jun 11, 2023
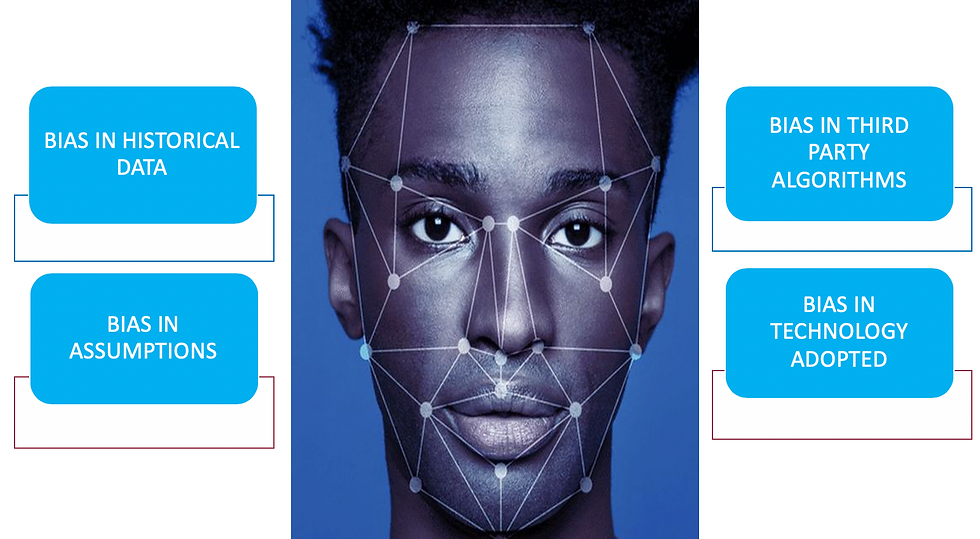
It is a known fact that poor design leads to unintended consequences of possible discrimination. As you build a platform that builds trust with your customers, what are some of the pitfalls that you need to avoid and sound design principles that you will adopt?
In a recent research published by Harvard Business School, Michael Luca, Associate Professor of Business Administration closely studies Airbnb - "If you're running an online marketplace," he says, "you should think about whether discrimination is likely to be a problem, and what design choices might mitigate the risk of discrimination. If you're optimizing only to short-run growth metrics and ignoring the potential for discrimination, you might be creating a blind spot."
For theboardiQ, a technology enabled platform with a moonshot to remove algorithmic and unconscious bias in the search, discovery, match, selection and onboarding of Inclusive Talent on to Board and Executive Roles, it is extremely important to prevent these blind spots!
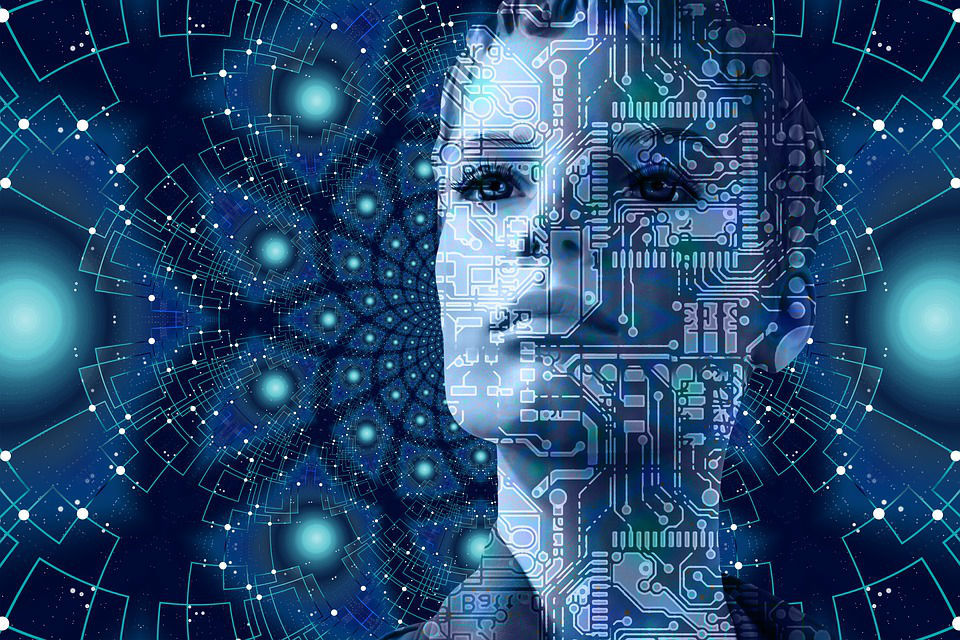
1. BIAS IN HISTORICAL DATA
Definition
This is the case when Algorithms are trained on historical data, to a large extent setting it up to merely repeat the past. If one wants to get beyond that, beyond automating the status quo, there is a need to examine the bias embedded in the data.
Inclusive AI done wrong - Case Study
Late 2018, Amazon’s machine-learning specialists uncovered a big problem: their new AI based recruiting engine did not like women.
Amazon’s computer models were trained to vet patterns in resumes submitted to the company over a 10 year period – most came from Men, a reflection of male dominance in the Tech Industry. Amazon’s system taught itself that male candidates were preferable – it penalized resumes that included the word “women’s” as in “women’s chess club captain.” And it downgraded graduates of two all-women’s colleges.
The dozen member Engineering Team set up at Amazon’s Edinburgh hub created 500 Computer Models focused on specific job functions and locations. They taught each to recognize 50,000 terms that showed up on past candidates’ resume.
The algorithms learned to assign little significance to skills and favored candidates who described themselves using verbs more commonly found on male engineers’ resumes, such as “executed” and “captured”
Consequence: The Program got scrapped and the team disbanded
Suggested recommendation to prevent bias: to adopt tools to detect and remove bias from algorithms – independent third party audits of algorithms – refrain from self certification.
2. BIAS IN ASSUMPTIONS
Definition
In the case of systems meant to automate candidate search and hiring - what assumptions about worth, ability and potential do these systems reflect and reproduce? Who was at the table when these assumptions were encoded?
Inclusive AI done wrong - Case Study
HireVue, creates models based on “top performers” at a firm, then uses emotion detection systems that pick up cues from the human face to evaluate job applicants based on these models. This is alarming, because firms that are using such software may not have diverse workforces to begin with, and often have decreasing diversity at the top. And given that systems like HireVue are proprietary and not open to review, how do we validate their claims to fairness and ensure that they aren’t simply tech-washing and amplifying longstanding patterns of discrimination?
Consequence: HireVue sought out third-party data-science experts to review their algorithms and methods to ensure they are state-of-the-art and without bias. However, they also reinforce the responsibility of the company itself to audit the algorithms as an ongoing, day-to-day process
Suggested recommendation to prevent bias: Although, HireVue passed on the responsibility of companies using its platform to ensure it is bias free, it does not absolve it from facing possible litigation and law suits on grounds of discrimination. It has to adopt tools to detect and remove bias from algorithms – independent third party audits of algorithms – refrain from self certification.
3. BIAS IN THIRD PARTY ALGORITHMS / DATA
Definition
Instances where bias is found in a third party’s algorithm, leading to discrimination in the hiring process, and a corresponding lawsuit follows - instead of having one applicant with a lawsuit, you would have many, because of how much the process has scaled. Companies may try to claim the third party as liable for damages, but that may not hold up in court.
Inclusive AI done wrong - Case Study
Facebook has been criticized in recent years over revelations that its technology allowed discrimination on the basis of race, and employers to discriminate on the basis of age. Now a group of job seekers is accusing Facebook of helping employers to exclude female candidates from recruiting campaigns.
The job seekers, in collaboration with the Communications Workers of America and the American Civil Liberties Union, filed charges with the federal Equal Employment Opportunity Commission against Facebook and nine employers.
The employers appear to have used Facebook’s targeting technology to exclude women from the users who received their advertisements. The social network not only allows advertisers to target users by their interests or background, it also gives advertisers the ability to exclude specific groups it calls “Ethnic Affinities.” Ads that exclude people based on race, gender and other sensitive factors are prohibited by federal law in employment.
Consequence: Facebook in March 2019, stopped allowing advertisers in key categories to show their messages only to people of a certain race, gender or age group.
Suggested recommendation to prevent bias: to adopt tools to detect and remove bias from algorithms – independent third party audits of algorithms – refrain from self certification.
4. BIAS IN TECHNOLOGY ADOPTED
Definition
Corporates as well as Law enforcement use a range of advanced technology to make their jobs easier, but facial analysis is one that is particularly powerful — and potentially dangerous. So-called "facial analysis" systems can be put to many uses, including automatically unlocking your Phone, letting a person into a building, identifying the sex or race of a person, or determining if the face matches a mugshot.
Inclusive AI done wrong - Case Study
Rekognition, Amazon's face-ID system, once identified Oprah Winfrey as male, in just one notable example of how the software can fail. It has also wrongly matched 28 members of Congress to a mugshot database. Another facial identification tool last year wrongly flagged a Brown University student as a suspect in Sri Lanka bombings, and the student went on to receive death threats. An MIT study of three commercial gender-recognition systems found they had errors rates of up to 34% for dark-skinned women — a rate nearly 49 times that for white men.
A Commerce Department study late last year showed similar findings. Looking at instances in which an algorithm wrongly identified two different people as the same person, the study found that error rates for African men and women were two orders of magnitude higher than for Eastern Europeans, who showed the lowest rates.
Repeating this exercise across a U.S. mugshot database, the researchers found that algorithms had the highest error rates for Native Americans as well as high rates for Asian and black women.
Consequence: Fairness and Facial Recognition tools available in the market need to train on a widely representative database of people - from different ethnicities, countries and color. Systems have to look at vast amounts of data to recognize the patterns within it.
Suggested recommendation to prevent bias: Some data scientists believe that, with enough "training" of artificial intelligence and exposure to a widely representative database of people, algorithms' bias issue can be eliminated. Yet even a system that classifies people with perfect accuracy can still be dangerous. Engaging independent third party audits of algorithms is critical – refrain from self certification.
Comments